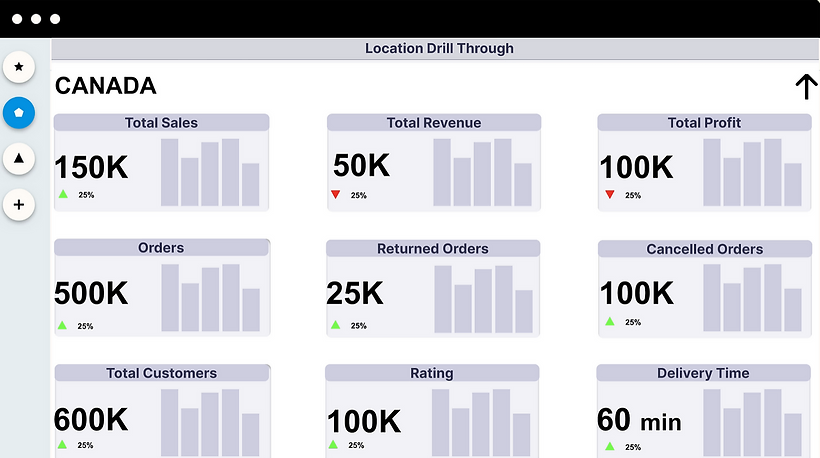
Manufacturing analytics, also known as industrial analytics, is the use of data analysis and machine learning techniques to optimize manufacturing processes and improve efficiency. It involves the collection, analysis, and visualization of data from various sources to gain insights into the manufacturing process and identify areas for improvement. While manufacturing analytics can help organizations gain a competitive advantage, there are several challenges that need to be addressed to achieve success.
In this blog, we will discuss some of the problems in manufacturing analytics and how organizations can overcome them.
- Data quality and quantity:
One of the major challenges in manufacturing analytics is data quality and quantity. Manufacturing processes generate vast amounts of data from sensors, machines, and production lines. However, not all data is relevant or useful for analysis. Organizations need to ensure that the data they collect is accurate, consistent, and complete.
The quality of data can be compromised by various factors such as human error, technical faults, and environmental factors. Additionally, there may be gaps in the data collected, leading to incomplete analysis. Organizations must have a data governance framework that ensures data quality and quantity.
2. Data integration:
Manufacturing analytics requires the integration of data from various sources such as sensors, machines, and production lines. However, different sources may use different data formats and protocols, making data integration a significant challenge. It is essential to have a data integration strategy that ensures the seamless integration of data from various sources.
3. Data security and privacy:
Manufacturing analytics involves the collection and analysis of sensitive data such as production schedules, machine configurations, and product designs. This data is often confidential and must be protected from unauthorized access. Organizations need to ensure that their data security and privacy policies comply with industry regulations.
4. Lack of expertise:
Manufacturing analytics requires specialized skills such as data science, machine learning, and statistical analysis. However, many organizations lack the necessary expertise in these areas. Without the right expertise, organizations may struggle to implement effective analytics solutions. It is essential to invest in training and development to build the necessary skills within the organization.
5. Resistance to change:
Manufacturing analytics requires changes in the way organizations operate. Some employees may resist these changes, leading to a lack of adoption and implementation. Organizations must communicate the benefits of manufacturing analytics to their employees and involve them in the implementation process.
6. Integration with existing systems:
Manufacturing analytics should be integrated with existing systems such as Enterprise Resource Planning (ERP) and Manufacturing Execution Systems (MES). However, integrating with existing systems can be a challenge due to different data formats and protocols. Organizations need to have a plan for integrating manufacturing analytics with existing systems to ensure seamless operations.
7. Cost:
Implementing manufacturing analytics can be costly, particularly for small and medium-sized enterprises (SMEs). SMEs may lack the financial resources to implement advanced analytics solutions. Organizations must have a plan for the cost-effective implementation of manufacturing analytics.
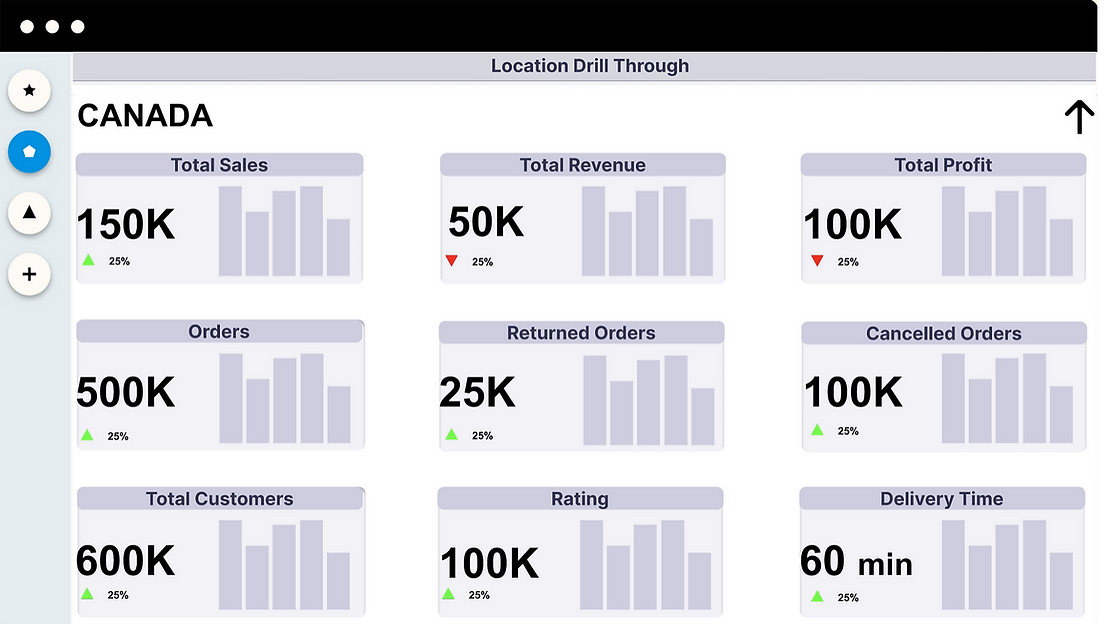
How to overcome the challenges in manufacturing analytics?
- Develop a data governance framework:
Organizations must develop a data governance framework that ensures data quality and quantity. This framework should include policies and procedures for data collection, storage, processing, and analysis. It should also define roles and responsibilities for data management.
2. Invest in data integration:
Organizations must invest in data integration to ensure the seamless integration of data from various sources. This may involve the use of integration platforms or the development of custom integration solutions.
3. Implement data security and privacy policies:
Organizations must implement data security and privacy policies that comply with industry regulations. These policies should define access controls, data encryption, and data retention policies. 4. Build expertise:
Organizations must invest in training and development to build the necessary skills within the organization. This may involve hiring data scientists, machine learning experts, and statisticians, or providing training to existing employees. Udshaa provides Manufacturing Analytics as a Dashboard-as-a-Service to help manufacturing organizations optimize their manufacturing productivity through correct and timely steps.